Home > Focus Areas > NSCLC Connect > Post
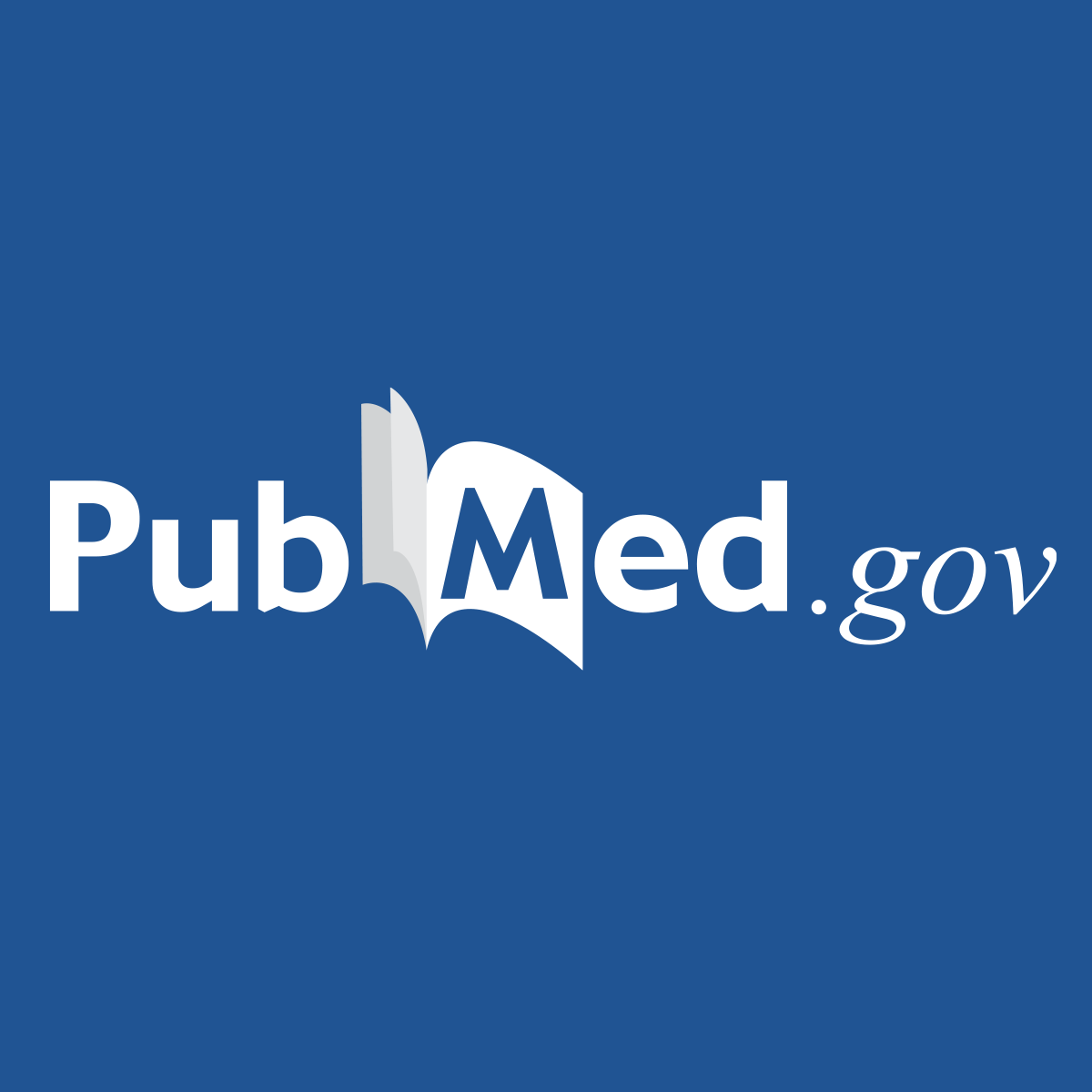
Multi-channel multi-task deep learning for predicting EGFR and KRAS mutations of non-small cell lung cancer on CT images - PubMed
Source : https://pubmed.ncbi.nlm.nih.gov/34079707/
1 School of Software, Taiyuan University of Technology, Taiyuan, China. 2 School of Information and Computer, Taiyuan University of Technology, Taiyuan, China. 3 Department of Radiology, Shanxi Province Cancer Hospital, Taiyuan, China.
Key Points
• As a means to provide targeted therapy, the prediction of the mutation status of EGFR and KRAS in NSCLC is useful. After all, CT is cost-effective and non-invasive. Deep-learning technology could help, however, it is difficult to apply due to small datasets.
• In the current study, researchers developed a multi-task deep learning (MMDL) employing multiple channels to characterize nodules and to integrate patient information into the process. The model was based on 363 cases, and the accuracy for EGFR-mutation prediction was % and that for KRAS was 72.25%. In 163 cases from The Cancer Imaging Archive (TCIA), the accuracy for EGFR was 75.06% and that for KRAS was 69.64%.
• The authors concluded, “Our method’s main advantage is that it is a non-invasive auxiliary detection method suitable for avoiding invasive damage if surgery and biopsy are not convenient. The acquisition of CT images is relatively inexpensive and fast. Moreover, our method does not require a physician’s domain knowledge, facilitating the economic and convenient prediction of gene mutations in EGFR and KRAS.”
• According to the authors, this model had certain limitations. First, the dataset was small and the data incomplete, with limited mutation data. Second, only 1 patient had both EGFR and KRAS mutations, thus it’s unclear whether the model could identify both mutations together. Third, the investigators did not use pathological studies. Fourth, the authors noted that “the effect of staging on the results was not reflected in our comparative experiments because it is not possible to conduct comparative